Data and analytics
Unlock the power of your data.
Data holds the key to improved outcomes and customer experience. But it may be locked in silos, inaccessible, inaccurate, or incomplete—slowing you down when you need to accelerate.
How do you empower your workforce and become a data-driven organization?
A strong data foundation opens a world of innovation and helps you make real-time, highly-informed decisions when it matters most—whether detecting infectious disease outbreaks, evaluating decarbonization pathways, or analyzing large genetic datasets to discover life-saving treatments.
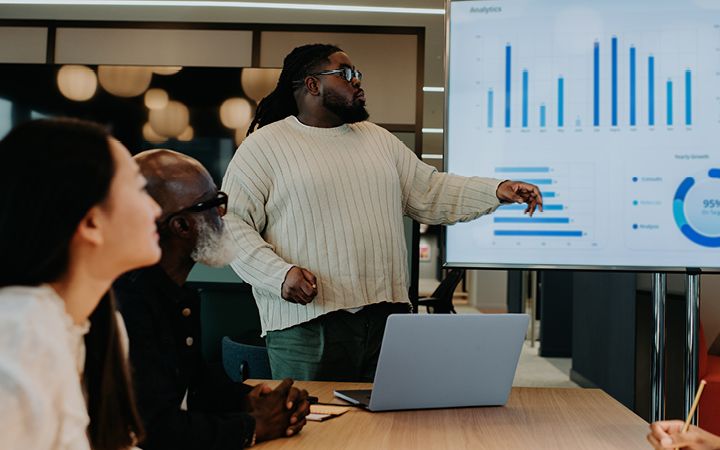
Our data modernization approach
To translate data into insights, you need a strong pipeline of accurate, high quality, and comprehensive data to power your analytics. That’s why we anchor our data solutions in deep domain expertise.
Our experts can work with your enterprise data—modernizing your infrastructure and developing data strategies that align to outcomes—or use publicly available or proprietary ICF data and technology platforms to help you make informed decisions.
We have 2,000+ public health experts and 2,000+ climate, energy, and environment specialists who understand your systems, policies, and regulatory frameworks. They know how to work with your data. And we pair them with data science and technology experts to accelerate meaningful solutions tailored to your context and mission needs.
200+
20+ years
4,000+
Our data and analytics services
Data and analytics strategy
Data governance
Predictive analytics
Fraud detection and prevention
Data skills training
Our work
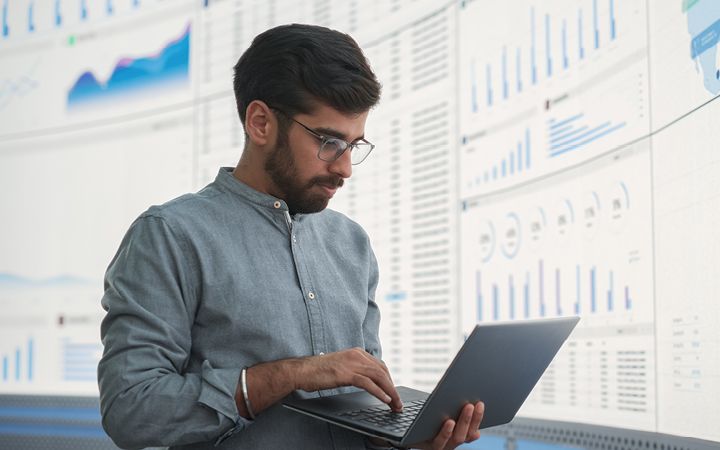
Develop consistent and repeatable data practices across your organization.
The secret to unlocking value from your data? Manage it like a product. We bring a data product mindset to our modernization work, creating high-quality, ready-to-use sets of data that anyone in the organization can access and apply to their business challenges.
Our experts
Related industries, services, and solutions