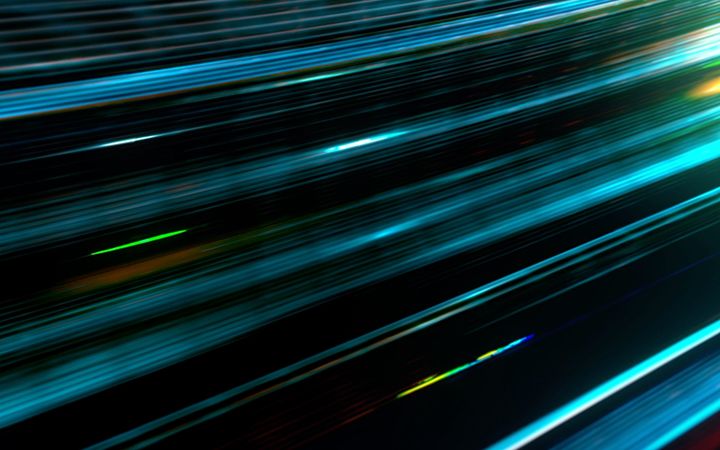
Introducing your newest teammate—generative AI
She recently graduated from college and is excited to apply all she has learned on the job! She’s extremely smart, super creative, and does a great job making connections. And did I tell you how fast she works?
I want to be honest, though. Your new teammate doesn’t have much real-world experience, and she’ll need your help learning the ropes. Make sure you provide lots of specific instructions and carefully review her work to correct for factual, logical, or grammatical errors.
Between your experience and good judgment, and gen AI’s knowledge and speed, I’m confident that, together, the two of you can do amazing things!
Caricature aside, much is being written about the concept of AI as a team member. While earlier technology innovations were seen as tools in our toolkit, AI is personified. For example, AI “learns,” “reasons,” and “hallucinates,” and now it’s “joining our team.” This concept can invoke mixed feelings of fear, skepticism, curiosity, excitement. What does AI bring to the table? How will we work together? Is this new team member a collaborator or a competitor?
One of the best ways to come to grips with these mixed emotions is to experiment and learn from experience. That lets us cut through the hype and learn specific lessons in the context of a particular use case.
We sat down with Joanne Barnieu, Lead Learning Scientist here at ICF, to learn more about her recent efforts experimenting with gen AI in the context of Learning & Development, and how her experience has informed her perspective on this idea of “AI as a Team Member” and its implications for the future of work.
How have you experimented with AI in your work?
I conduct research projects that incorporate the use of natural language processing. Recently, I was also able to experiment with AI to analyze feedback provided by training participants. Open-ended survey comments often elaborate on what participants liked, didn’t like, and learned from the training, and while this data is useful, it often goes unanalyzed because organizations don’t have the time to perform analyses of feedback from hundreds or thousands of training offerings.
So our team wondered, would AI produce similar results to a human when analyzing these comments? If so, could organizations benefit from insights into this otherwise-untapped data source? We designed an experiment to test these questions by comparing my own, manual analysis of the comments with AI-generated results.
What were the outcomes?
First, we needed to see if AI would produce usable themes from the survey feedback. After reviewing the initial themes produced by AI, our honest assessment was that while some were useful, others were too vague. For example, a theme such as “Course Quality” would not sufficiently capture nuances to categorize comments in a meaningful way.
Second, we wanted to see if AI properly associated data with a particular theme. For example, did the AI take a comment like “How to talk to people and have them open up” and align it to a theme like “Communication”? To test this, we provided AI with human-generated themes for feedback on two evaluation questions. We found two key things: The AI was more accurate when there were fewer themes, and the AI was about 60% - 70% accurate compared to human analysis.
What did you notice? What were some of AI’s strengths and limitations?
Overall, “curiosity” is the best word to classify my experience. As someone who has done many thematic analyses, I was open to seeing how AI could tackle this task. If nothing else, being part of the experiment helped reinforce my understanding of what AI can and can’t yet do.
As I’d suspected based on my own experience and research, AI was able to accomplish the task much faster than a human, but it wasn’t without error. Accuracy can likely be improved over time with better prompting or even fine-tuning of the model, but for now, human review is still needed.
The results made me reflect on a few other factors that might contribute to accuracy in this context, whether the analysis is undertaken by AI or a human reviewer:
The data you receive is only as good as the questions asked. Both humans and AI will struggle to identify common themes for wide-ranging responses to poorly worded or overly broad questions.
Questions on a training course evaluation can lend themselves to multi-part answers. AI may struggle more than a human to consistently tease out the various parts of the answer into different themes. These responses can be improved with more thorough prompting, but that may require an experienced partner’s prompt engineering expertise to fully solve.
Some respondents provided short answers of limited value. For example, when asked what part of course is most helpful, they might answer “all.” While “all” is favorable in this context, neither humans nor AI can easily classify the comment into a particular theme.
Ultimately, even though the AI didn’t perform perfectly, it provided a great starting point for further refinement. And by combining AI’s analysis with human review, we were able to achieve similar results in a fraction of the time for an otherwise unused data source. That’s a real benefit.
Looking ahead, how do you envision AI transforming your work?
I see AI helping to reduce human effort without entirely replacing a person. Using our experiment as an example, I think AI can help produce and analyze the frequency of usable feedback themes as long as the themes and results are reviewed and edited by a human. Eventually, AI models may be fine-tuned to improve theme prediction, further reducing the level of effort.
Applying AI to something like course evaluations is just the beginning. This new “team member” can apply to any type of qualitative data analysis, which we do so much of in the field of workforce research. And if we can reduce the time spent on analysis, we can get to insights, action, and results much faster.