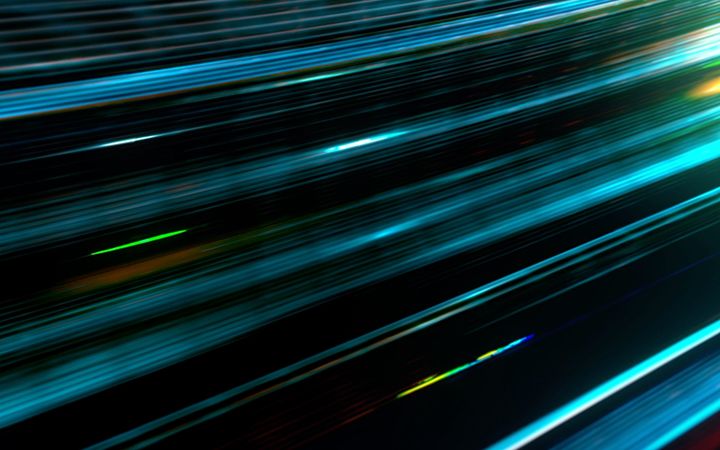
Can you really rely on big data for program and policy evaluation?
Big data receives an enormous amount of hyperbole about the potential advantages for evidence-based policymaking. It can widen the scope and value of project and program design and subsequent evaluation. But, this process is not without risks. By its nature, big data entails quantity but variable quality—demanding integrity, skill, and care to apply it effectively. Without caution, it can point policymakers in the wrong direction.
Where does big data come from, and why do we hear so much buzz about it?
Big data refers to a vast amount of digital information that can be collected across a range of sources: mobile phones, the internet, surveillance cameras, environmental sensors, and social media. It is often divided into three categories:
- social data
- observed data, and
- transactional data
The breadth of this information is enormous and complex. It requires new ways to manage, store, and analyze data. So, despite much buzz, it’s still a developing science.
The hype cycle surrounding big data moved much faster than the maturity of the technology itself—like it often does when a breakthrough hits the market. This cycle suggests there is a period of exaggerated expectations where early success stories can eclipse the failures. Marketers selling the technology tend to make brash promises that it may subsequently fail to deliver. Though big data became the hottest topic of media buzz before it was ready, we are now entering a time when—if used correctly—it offers tangible benefits for evaluators and policymakers.
To make big data a powerful tool for your evaluation, keep in mind these four essential recommendations.
1. Combine big data with traditional research methods
Evaluators who want to assess the extent to which a project, program, or campaign meets its original objectives can use big data as a helpful and cost-effective resource. But, it’s often not enough to draw conclusions—you need to apply traditional research methods for a full picture.
An arresting illustration of this comes from a 2009 story in Rwanda, where a research team measured how wealth was distributed geographically across the country.
Researchers conducted a survey of 1,000 people who were randomly selected from a database of 1.5 million registered phone users. In addition to the survey data, the researchers had access to the phone records of 1.5 million customers. Combining these phone records with the survey findings enabled the team to train a machine learning model to predict a person’s wealth based on their call records.
The model was then used to estimate the wealth of all 1.5 million people in the database. They also used geographical information embedded in the phone records to estimate where each person lived. The overall outcome was the creation of a high-resolution map of the geographical distribution of wealth within Rwanda.
Although the findings could not be validated against other sources, aggregated findings bore a very close relation to ICF’s work on the Demographic Health Survey (DHS) for the U.S. Agency for International Development (USAID). The latter is considered as the gold standard for surveys in the developing world. Identifying this correlation doesn’t mean the regular DHS is no longer necessary but the combination of these two sources enabled researchers to make more efficient use of resources. Key issues that needed to be covered through a survey could be prioritized, leaving others to come from other readily available, unstructured sources.
This innovative approach of combining big data with more traditional research methods has proved invaluable in more recent program and campaign evaluations where data from traditional survey and focus groups was combined with readily available unstructured, big data.
2. Keep an eye out for misleading patterns
Not all types of data lend themselves well to big data analysis. Often, qualitative and less tangible measurements are unsuitable. ICF’s evaluation team discovered one such limitation while analyzing the Erasmus+ program in Europe.
We sought to assess the relevance and visibility of the program, collecting—amongst other aspects—the volume of social media posts about Erasmus+ and the sentiments connected with it. In addition, the team analyzed the audience of social media users, their demographics, and location.
More than 750,000 posts were processed over a year in English, French, Spanish, and German. The sources used to identify content on social media were Twitter, Facebook, and Instagram.
The analysis showed that the most used language was Spanish, and most users communicated in their native language. So, multilingual communication was crucial to reach larger audiences. The analysis also showed that social factors played a significant role in the sharing of topics.
But the evaluators encountered a problem regarding the sentiment analysis that highlighted the limits of artificial intelligence and big data. The program assessed every post connected to Erasmus+ and decided whether the post was negative, neutral, or positive. It sometimes misjudged the sentiment, especially when the user used complex sentences, jokes, or sarcasm.
For example, the team observed very strong negative sentiments connected to Erasmus+ in the UK. A deeper analysis showed that the negative sentiment was, in fact, not directed towards Erasmus+ but rather to concerns about the continuation of the program after the Brexit referendum.
As this example shows, you need experienced evaluators who can unearth misleading patterns and find the correct explanation.
3. Foster collaboration between data scientists and evaluators
There is an argument in favor of data teams and evaluators working closely together in setting up projects, programs, and campaigns. This is particularly important when it comes to research methods, as many data scientists do not have the training and background in conventional evaluation methodology. Similarly, evaluators may not see the value that data scientists bring unless close links are forged. If these specialists stay in their own silos, the dangers inherent in using big data may not be recognized, so mistakes can be introduced inadvertently.
4. Understand the difference between 'analysis' and 'analytics'
‘Analysis’ and ‘analytics’ are often used as interchangeable terms. However, they do have subtly different meanings. ‘Analysis’ covers assessing information for outcomes in the past. The term ‘analytics’ describes predictive analysis, which is where big data can come into its own. Machine learning can provide insight and understanding quickly, so real-time learnings are fed back rapidly into the research methods before the research program, project, or campaign is over. The potential for making the most of the data and findings is thus heightened.
The strengths and limitations of using big data in evaluations
Big data can provide additional, unique insights that give a much more comprehensive picture than traditional methods alone. Key benefits include:
- Objective measurement outcomes
- Analytics can be near real-time
The role of big data is set to increase in the foreseeable future. It can be successfully integrated into evaluations to strengthen the evidence of the effects of a program. It has the benefit of handling massive amounts of information quickly, enabling greater insight in real-time so interventions can be optimized shortly after launch.
We cannot overemphasize the importance of an evaluator’s skill, knowledge, or ability to bring order, coherence, and value to the use of big data. Initially, it is just raw, unstructured information that needs refining. It certainly adds a new dimension and greater insight into research when used alongside traditional survey methods. But just like traditional survey design and evaluation, the same principles apply: you need skilled project management, a well-defined strategy, clear project scope, and excellent understanding of how to run an evaluation appropriately. Managed judiciously—and with respect for issues of privacy and individual rights—the potential of big data is endless.