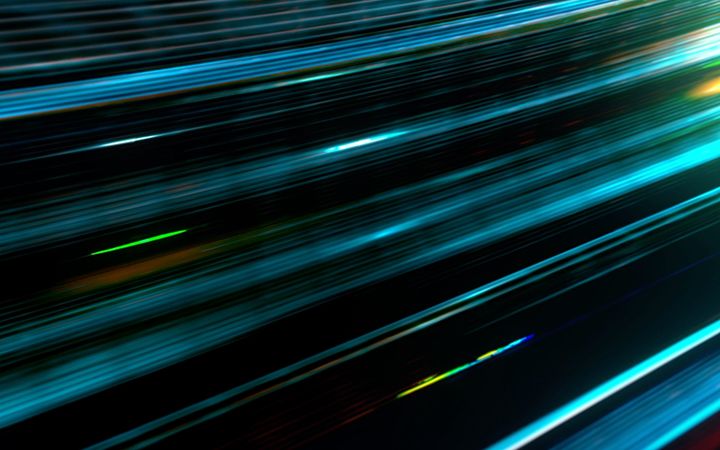
How to get your agency data ready for AI
Federal agencies have an abundance of data that needs to be ready for both everyday artificial intelligence, such as increased personalization and assisted work tasks, and transformational artificial intelligence, such as automated data analysis. But with so much data, how can it be made AI-ready in a safe, trustworthy, and cost-effective way?
Based on our years of work using both generative AI (Gen AI) and more established AI, along with our recent work for the National Institutes of Health (NIH), we’ve compiled four things to consider when evaluating datasets for AI-readiness—and why it’s essential for mission delivery in this new age of AI-powered government.
Identify your challenge
Our team recently won the NIH National Institute of Diabetes and Digestive and Kidney Diseases (NIDDK) Data Centric Challenge, aimed at preparing their datasets for future AI applications. We normalized several data elements and addressed missing values that could affect analysis for NIDDK, solving for multiple applications—including predictive models for identifying risk factors and outcomes related to Type 1 diabetes and understanding the development of diabetes.
Before you begin to prep your data to leverage AI, ask yourself: What specific problem am I solving for? Most AI use cases fall into one of three categories: User experience, workflow, and key operations. Some challenges Some challenges are data-intensive, in which case the volume, quantity, and quality of data matters, like when FDA used AI to streamline their drug safety review process. But others don’t need a large volume of to solve—such as clinical decision support systems, when healthcare providers use AI to make recommendations based on clinical guidelines but don’t require an abundance of past data. Identifying your challenge ahead of time will help ensure you get the right data ready for AI without spending time on unnecessary data.
Learn about new data quality dimensions
Data guidelines that worked before, such as FAIR principles (Findable, Accessible, Interoperable, Reusable) may not be sufficient for AI-readiness. Instead of relying on them wholly, make sure you apply ACCR as well: Accuracy, Completeness, Consistency, Relevance.
While FAIR principles are well-established in the data science community to guarantee the accessibility and usability of data, the concepts of accuracy, completeness, consistency, and relevance are commonly considered essential for ensuring the data is AI-ready. For example, ensuring data accuracy helps prevent errors in AI models, while completeness ensures that all necessary information is present, and relevance ensures that the data aligns with the specific objectives of the analysis. By applying these principles alongside FAIR, agencies can ensure that AI algorithms are built on high-quality data leading to more reliable and actionable insights.
Work for machine-readable data
Artificial intelligence in all its forms and iterations, including Gen AI, is a machine, and machines need standardized formats and dictionaries. AI cannot read or interpret data that is unconventionally formatted or uses unfamiliar terms, such as faxes, lab work, metadata, and notes. That doesn’t mean all those documents and data types are unusable—it just means that data needs to be organized by a human to make sure the AI is reading and analyzing it correctly and producing outputs that are as accurate as a human would. Once your people are trained to do that, checking that data is machine-readable should be an essential early step to the continual analysis process.
Aim for explainable AI
When working with AI, you need more than just transparency into the data it’s using—you need the entire process, and each output, to be explainable. That will ensure responsible and ethical data use and create a solid foundation on which to build further analyses and iterate new models for data processing as AI builds up from the everyday to the transformational.
As agencies get ready for AI, either for the first time or to increase their adoption, they need to do so with confidence and security while remaining cost-effective. Gen AI may be new, but it builds on the AI and data science we’ve been working with for years. Partnering with a team that has combined data, emerging technology, and federal program experience can help guarantee agency transformation and mission success with proven data modernization and AI solutions custom-built for your unique needs. Once the data is ready for AI, agencies can benefit from faster data analysis, greater data accessibility, deeper and broader insights, and a foundation that can be built upon as technologies continue to innovate.