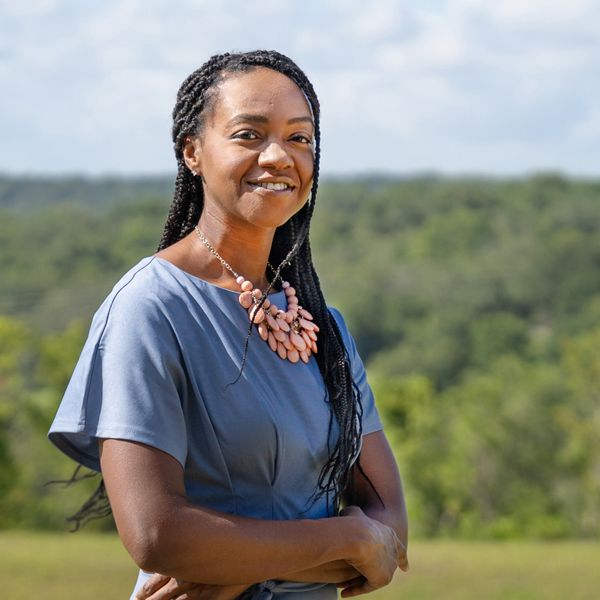
INSIDE ICF
Q&A with Rachel Alexander
Series: Powered by purpose
What if a prescription label could prevent an illness?
"We're harnessing the power of artificial intelligence, machine learning, and computer vision to solve real-world, potentially life-threatening challenges."
Rachel is using cutting-edge technology to develop a prototype that streamlines and minimizes inefficiencies in the drug labeling review process. Her goal? To reduce the number of adverse reactions and accidental deaths caused by misinterpreted labels.
Q: What do you do?
A: I’m leading a pilot project with the U.S. Food and Drug Administration (FDA) called the Computerized Label Assessment Tool, or CLAT. We’re using technology—including machine learning and artificial intelligence (AI)—to make the process of reviewing prescription drug labels much more efficient.
Q: Why is this important?
A: Since 2013, there have been over 1,600 cases of serious injury or death related to drug label errors reported to the FDA. These labels are the FDA’s primary tool for communicating medication information. So, when they are unclear, people can actually get sicker or even die. Improving the prescription labeling process helps doctors, nurse practitioners, physicians, pharmacists, and patients avoid grave errors.
And right now, the process is highly manual and labor- and time-intensive. Typically, a reviewer performs 25 to 50 drug label reviews every year, analyzing everything from a label’s font size to word placement. And that’s just one reviewer.
Our prototype uses some of the industry's leading optical character recognition and object detection tools to expedite and streamline this process for drug labeling reviewers—with the ultimate goal of benefiting all healthcare consumers. We help answer questions like: If a medication is made in multiple strengths, are its labels easy to differentiate? Or could someone take the wrong dose because the labels are too similar?
On a daily basis, this project could impact tens of thousands of lives.
Q: How did you look at this problem differently?
A: CLAT is a tool I helped build that uses algorithms and machine vision to read drug labels and pinpoint specific items for examination by the human reviewers.
Through machine learning and highly advanced algorithms, CLAT gets more and more accurate in recognizing and classifying thousands of data sets and label images. Our team also makes recommendations on standard images that could represent a particular drug type—for example, the same universal icon of an ear on all drugs that help with ear-related issues. This makes the FDA review process easier and reduces confusion among patients and caregivers.
Q: What’s the next step?
A: There are hundreds of thousands of drugs on the market, so we’ll continue to gather data through AI-based reviews and mature our processes. With better data, we’ll be able to provide better input for CLAT’s machine learning. The more we feed it, the more accurate the models get. This will, hopefully, lead to exponential jumps in efficiency. And the FDA continues to explore how this machine learning prototype could be used in other review processes, which is gratifying.
Q: How does this project connect to your purpose?
A: I enjoy challenging the way things have always been done. And as Black woman in the technology industry, I'm motivated to drive progress. It’s important to me to show that taking chances and calculated risks can pay off. Which is exactly what I’m able to do with CLAT.
This project is the first of its kind at both ICF and the FDA to harness the power of artificial intelligence, machine learning, and computer vision to solve real-world, potentially life-threatening challenges. This has given me the freedom to think outside the box and innovate in unconventional ways. I’m grateful to be able to push the boundaries of what is possible, while making tangible impact.